Intel’s new Loihi-2 chip uses 1 million neurons
The second version, which has just been released, shows improvements in all areas.
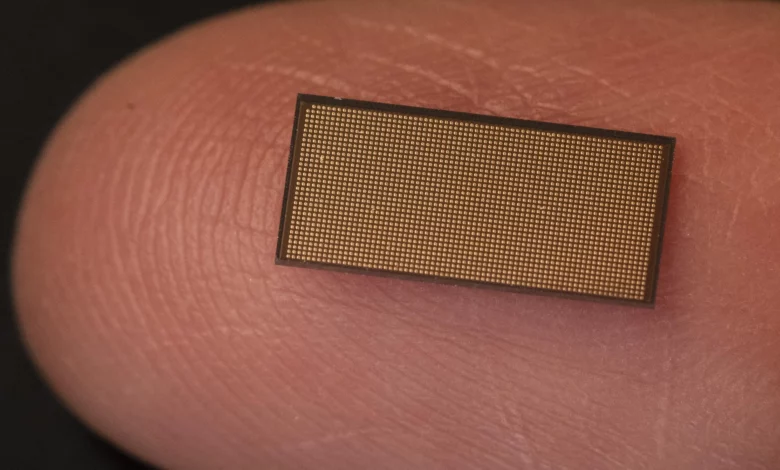
The Loihi 2 is smaller, more efficient, and faster than its predecessor, thanks to brain-enhancing Intel chip enhancements. The first version of the chip, designed for so-called neuromorphic computing, was introduced in 2017. The second version, now released, shows improvements in all areas as Intel has significantly improved its architecture and manufacturing.
While the Loihi was manufactured using the 14nm process, the Loihi 2 is based on a pre-release version of the upcoming Intel 4 (formerly known as the 7+nm EUV) technology. The chip’s surface area is 31 nm instead of 60 nm, which means that the structure has been reduced by half, but it still has one million neurons compared to 128 thousand.
The internal structure contains 128 neuronal nuclei in a two-dimensional 8×16 mesh, eight times the number of neurons. An additional 128 Kbyte of local SRAM is available for each core, but this can now be flexibly partitioned. The new compression also has excellent benefits, for example, in the state of neurons or axon routing.
Off-chip communication is also essential as Intel uses the Loihi 2 on multiple systems. Therefore, instead of 2D, a 3D array is used, which together with the compression of the message is said to increase the speed tenfold. For the time being, Intel is offering the Loihi 2 as a chip development board (Oheo Gulch), followed later by a version with eight neuromorphic computing processors (Kapoho Point).
Because the hardware alone is of little use, Intel has also upgraded the software in parallel with the Loihi 2. The Lava framework was created from the previous Nx-SDK (Software Development Kit) and will be available as an open-source license in the future (BSD-3 / LGPL-2.1 license) on Github, for example.
Among other things, Lava integrates a Python interface and can be used on CPU / GPU platforms, which significantly simplifies development. Frameworks like Nengo, Pytorch, Robot Operating System (ROS), Tensorflow, or Yarp are also easy to integrate.